Neural networks are powerful approximators, capable of capturing the complex behavior of real-world phenomena that are difficult to describe explicitly. This capability is being exploited across many scientific domains such as molecular biology or materials engineering. A trained network used in these applications can be viewed as a solver optimized for a specific domain, limiting its applicability to others. The "holy grail" of artificial intelligence is to design a general-purpose problem solver capable of addressing arbitrary domains. Recently, large language models have demonstrated impressive general-purpose problem-solving abilities by imitating language behavior, which can be seen as a product of what is arguably the most general-purpose problem solver we know of. However, the mechanisms underlying these models remain poorly understood. This workshop focuses on research directions that contribute to understanding the general-purpose problem-solving abilities of neural networks.
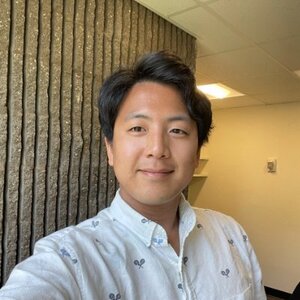
Core Francisco Park
(Harvard)
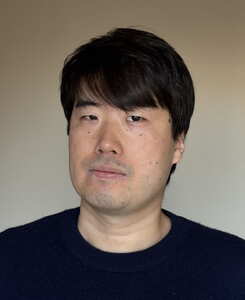
Kazuki Irie
(Harvard)
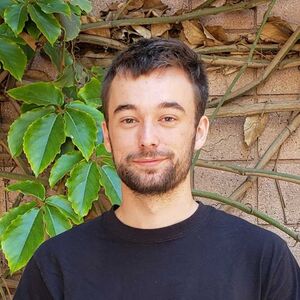
William T. Redman
(Johns Hopkins)
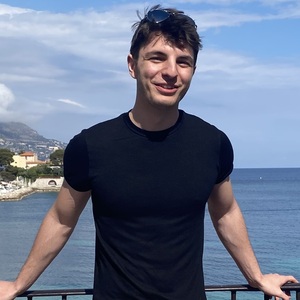
Federico Barbero
(Oxford)
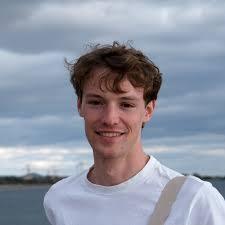
Jonas Hübotter
(ETH Zurich)
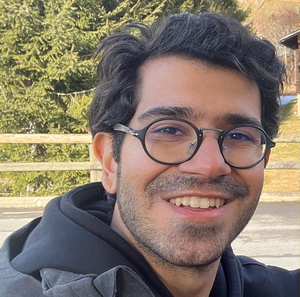
Aryo Lotfi
(EPFL)
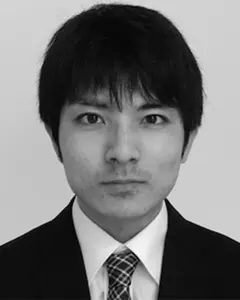
Takeru Miyato
(U. Tübingen)
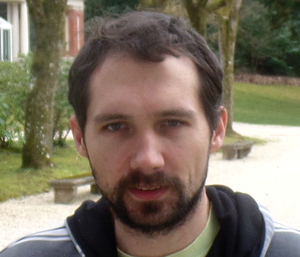
Mirek Olšák
(Oxford/DeepMind)
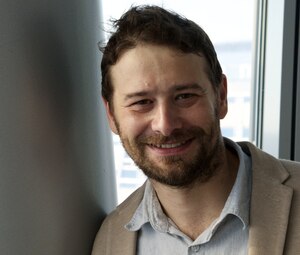
Mikoláš Janota
(CIIRC)
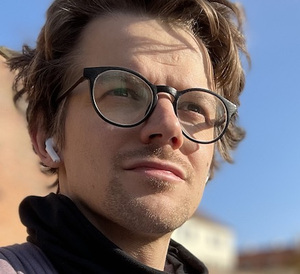
Vít Musil
(MUNI)
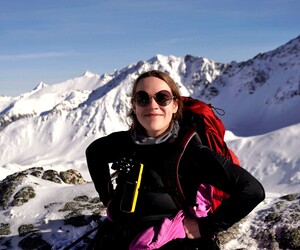
Alicja Ziarko
(IDEAS NCBR)
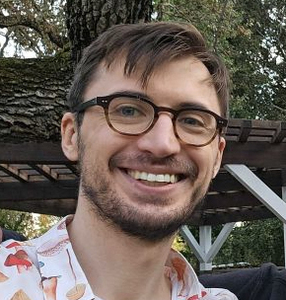
Gracjan Góral
(IDEAS NCBR)